1.1 Rigorous Open Science
Less tangible, but not less important for the perceived veracity of scientific knowledge are concepts such as intellectual honesty and scientific integrity of the scientists laying explanatory claims on some domain in reality. Merely checking whether the scientific method has been applied does not fully grasp all the prerequisites for generating a solid body of knowledge. We will use the term rigorous open science to denote the ideal set of conditions that should be in place to allow us to distinguish scientific claims that are likely to be false, from claims that are likely to be true, given the perceived verisimilitude (truth-likeness) of the knowledge accumulated in the scientific record.
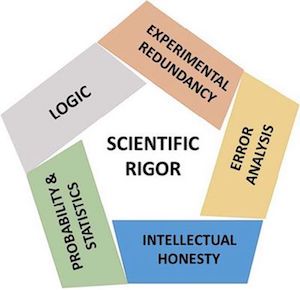
Figure 1.1: Rigorous Science according to Casadevall & Fang (2016).
When a claim is based on Scientific Rigour (Casadevall & Fang, 2016), we mean it was posited based on the following set of principles:
- Experimental Redundancy - The claim has been examined by all methodological and analytical tools that are available and are appropriate given the context. Rigorous Science does not rely on one type of experimental design or one type of statistical analysis.
- Recognition of Error - Without failure there can be no progress, therefore we should carefully study failures and not just report success stories. Any sources of error should be carefully studied and reported to the scientific community.
- Sound Probability & Statistics - Use of the most recent and appropriate statistical theories, models and analytical techniques. Statistical modelling techniques become more realistic over time and often the models that were taught in undergraduate statistics courses have long been replaced and should not be used any more.
- Efforts to Avoid Logical Traps - When generating theories and defining constructs and laws, make sure logical inconsistencies are avoided. When making inferences, avoid the common logical traps such as The Effect = Structure Fallacy in null hypothesis significance testing (NHST).
- Intellectual Honesty - Rigorous science is ethical, has integrity and thrives on critical reflection on scientific practice. The right mindset is “Prove yourself wrong!”, not “Prove yourself right!”
We add to the list that science must be open and transparent. This may seem like an obvious statement to a fresh student of human behaviour, but concepts that make up an essential part of the scientific debate in 2017, such as open science, open data, reproducibility, Questionable Research Practices (QRPs), Hypothesizing After the Results are Known (HARKing) and preregistration, were practically unknown 5 years ago.
Comedian John Oliver discusses how and why media outlets so often report untrue or incomplete information as science:
Strong Inference
A difficulty of much psychological theorizing is vagueness in the terms employed. In this work, the above ideas have been studied in mathematical form throughout, the definitions and proofs being given corresponding precision.
—W. R. Ashby in ‘The Physical Origin of Adaptation by Trial adn Error’ (1945, p. 13)
The Effect = Structure Fallacy refers to the logical error that occurs a predicted effect is observed (i.e. a statistically significant test result leads to a rejection of the null hypothesis), it is not valid to infer the existence of the assumed cause was evidenced. NHST is based on the falsification principle, which means the perceived veracity of a scientific claim will increase only if it has resisted many rigorous attempts to prove it is wrong. If a scientific claim has a large track-record of resisting falsification attempts, we can call it plausible, or high in verisimilitude, but this could all change with one crucial experiment. Contrary to what some scholars suggest, falsifiability is not optional in a rigorous science (LeBel, Berger, Campbell, & Loving, 2017).
An excellent recipe for a rigorous application of the scientific method was provided by Platt (1964). Perhaps we should implement it and get us out of the curious situation in which so many different “theories” competing to explain the same pehnomena can be considered to be “true” at the same point in time.
Strong inference consists of applying the following steps to every problem in science, formally and explicitly and regularly: | |
---|---|
|
Devising alternative hypotheses |
|
Devising a crucial experiment (or several of them), with alternative possible outcomes, each of which will, as nearly as possible, exclude one or more of the hypotheses |
|
Carrying out the experiment so as to get a clean result |
1’ | Recycling the procedure, making subhypotheses or sequential hypotheses to refine the possibilities that remain |
… | and so on. |